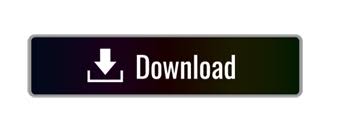

» » » Is it a convergence/optimizer thing? Sounds counterintuitive first, but it happens. » In what cases we do observe an intersubject variability is less than intrasubject variability?Īnd I answered that I have no idea why. What was the question (see subject line)? » » And would it in SAS be something other than PROC GLM cf. This is implemented in Bear I think, see ANOVA_stat.txt "prob: the probability for obtaining a negative estimate of inter-subject variability". Don't remember the chapter number exactly. using the least square optimizer in fitting the model with all effects fixed). Or what is meant here?īTW: Liu & Chow have a chapter dealing with negative between-subject variance in the context of ordinary ANOVA (i.e. Maybe the WinNonlin doesn't obey this reasonable rule? And therefore the between-subject variance may come out as negative. At least in SAS this is the default setting which must be overred if you want an unconstrained solution. Using ML or REML usually implies that variance-covariance terms are fitted with the constraints that they must be >= 0. Meanwhile I think it is an optimizer thing. If you give me some days I will dig out a data set.*Ĭan play with the data if my SAS is again in reach to me. » Sequence+Subject(Sequence)+Formulation+Period Probably a more appropriate model is to move Subj(Seq) out of the random model and into the fixed model, i.e., » The negative final Variance Component warning most likely indicates that, if using Subj(Seq) as a random effect, the within-subject variance (residual) is greater than the between-subject variance.
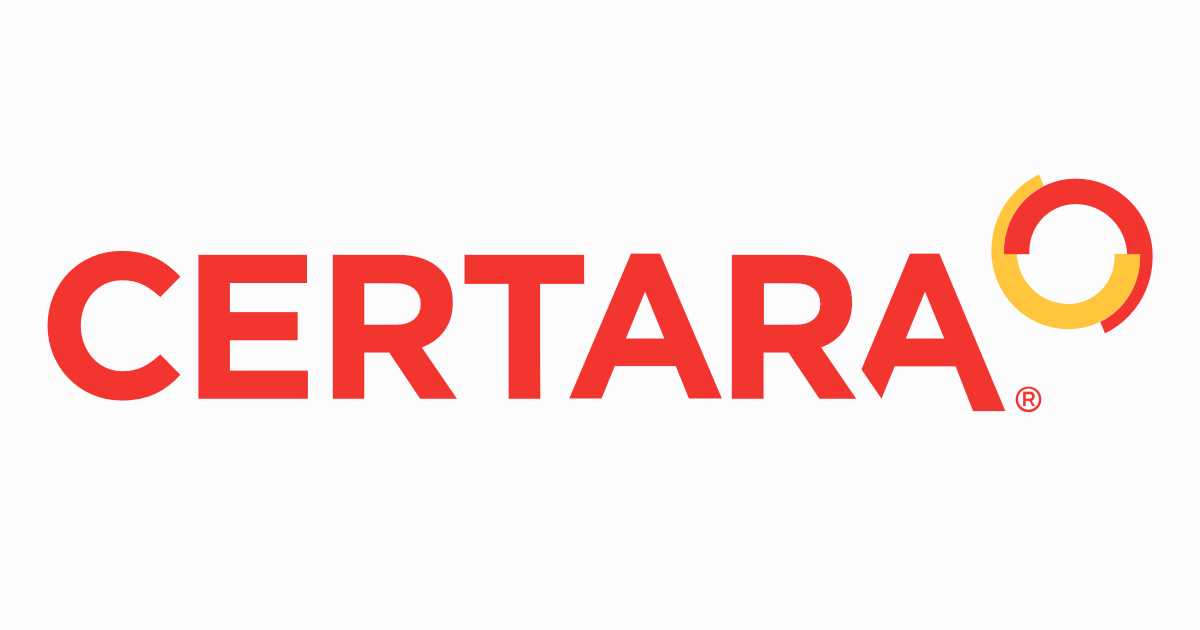
I am not extremely well versed with either package but am curious to learn why this would need be done. Somehow surprising since the individual T/R-ratios range from 19.9–365%! PHX could fit subjects(sequence) as random without any tweaks. Folinic acid after 15 mg IR calcium folinate, SD, fasting, LLOQ 5 ng/mL (0.95% of C max), all extrapolated areas <20% (median 6.9%), n=24 (n RT=11, n TR=13):
WINNONLIN OPTIMIZER FULL
The full results of the comparison and the model files in SAAM II and PCNonlin/WinNonlin formats are available from the authors.» Interesting. Additionally, there were differences when multiple data sets were fitted, indicating the importance of different fitting procedures for interpreting multiple kinetic data sets. However, due to differences in the optimization procedure, parameter standard errors showed considerable differences. In general, there was good agreement (<1% difference) between SAAM II and PCNonlin in terms of parameter estimates and model predictions. Observed differences, mainly in parameter standard errors, can be accounted for in terms of different optimization algorithms, convergence criteria, and individual capabilities. Parameter estimates, their precision (standard errors), and model predictions were compared a difference of 1% or less was considered “agreement”. The total number of attempted comparisons between SAAM II and PCNonlin was 161, of which 142 executed without problems. We compared 88 different models, many of them in different configurations, e.g., different weighting schemes or different parameter limits. Maximum number of compartments, data sets, and parameters were 9, 5, and 10, respectively. Models investigated included one- and multicompart-ment models with nonlinearities, multiple inputs and samples, multiple simultaneous experiments, and linear equations. Models were initially executed in PCNonlin or WinNonlin and automated comparisons with SAAM II made using Microsoft Test.
WINNONLIN OPTIMIZER SOFTWARE
For each model, both software packages were presented with identical implementations. This paper presents a detailed comparison of the kinetic analysis software packages SAAM II and PCNonlin/WinNonlin, based on benchmark modeling problems reported in “ Pharmacokinetic and Pharmacodynamic Data Analysis: Concepts and Applications” (Gab-rielsson and Weiner, 1994) and seven additional models.
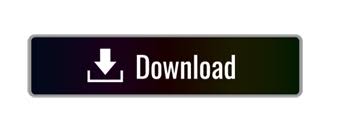